Blind charging
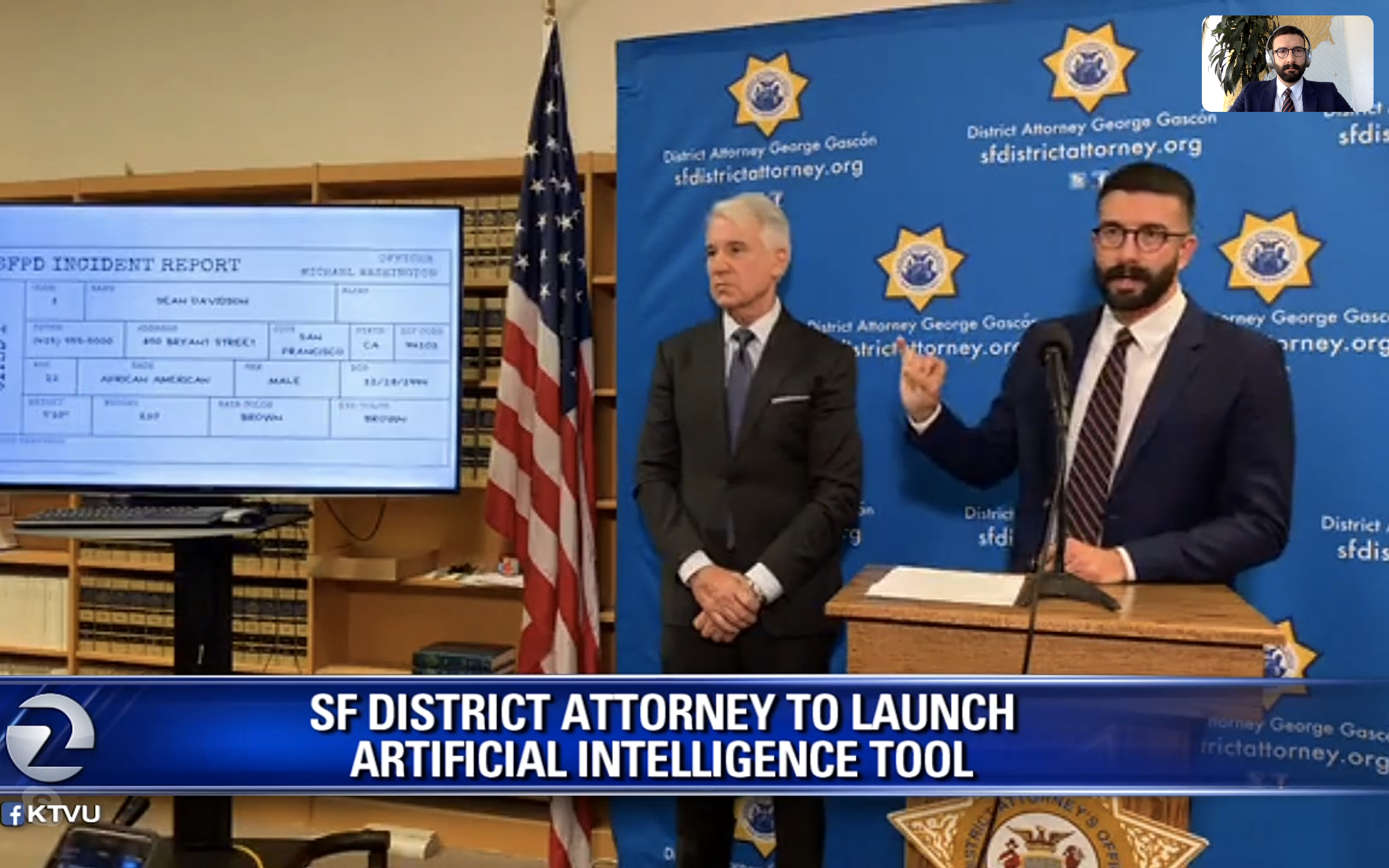
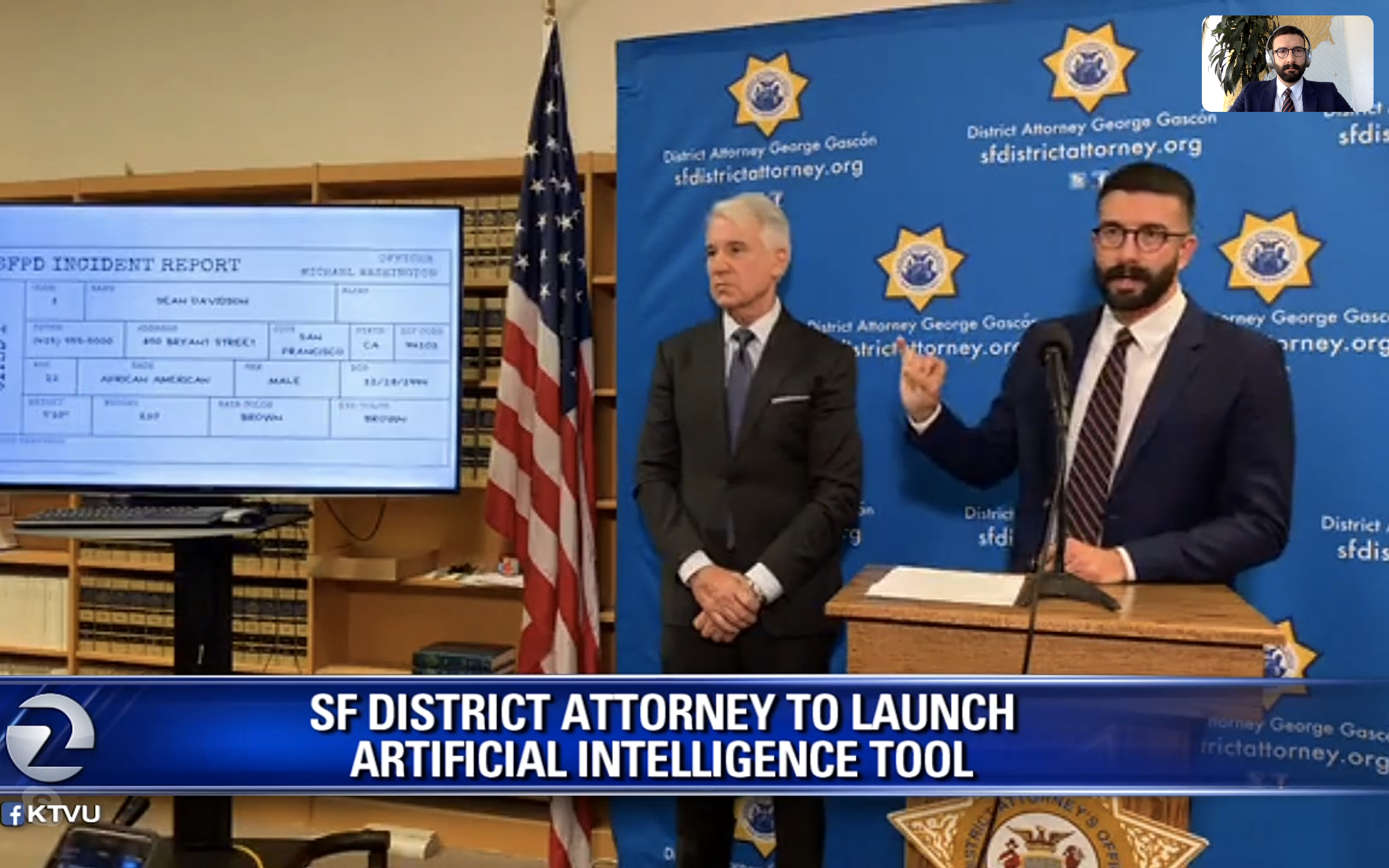
With colleagues at CPL, I designed an algorithm that uses LLMs to automatically mask race-related information in police reports.
Prosecutors then review these redacted reports and make a race-blind decision to charge or dismiss each case.
After we ran pilots at the San Francisco and Yolo District Attorney’s offices,
California passed a law
requiring prosecutors across the state to adopt our intervention.
We are now studying the impacts of blind charging with a randomized controlled trial.
Blind charging has been covered in numerous press articles.
Learn more at blindcharging.org.